Solution
Analytics
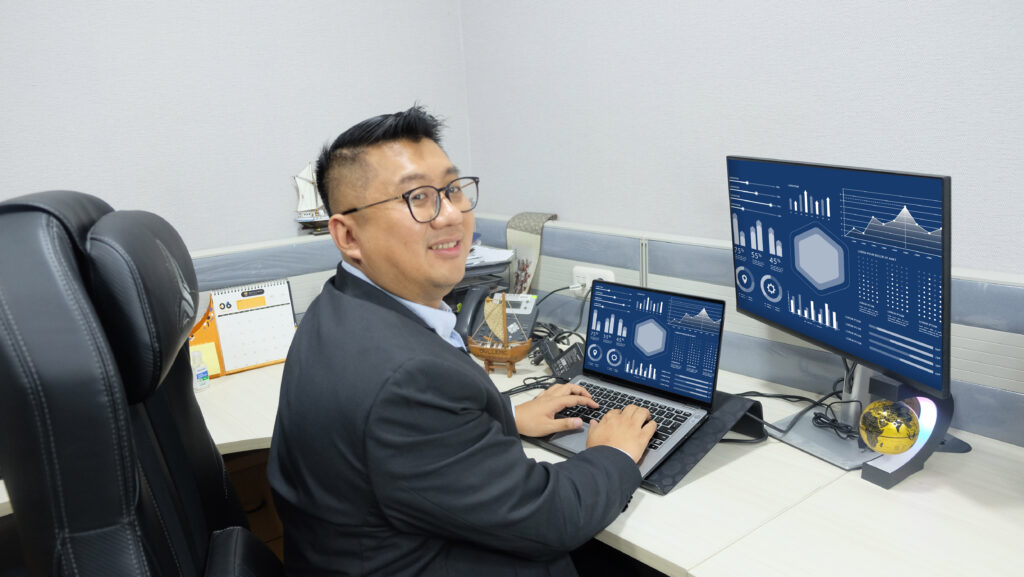
Self Service Analytics for Data Lakehouse
Self Service Analytics for Data Lakehouse :
In the data-driven era, you need to be able to generate value from all of your data capital, from edge to multiple clouds to core. Yet, because data is scattered, doing so can be difficult and expensive. This creates a barrier to insight and innovation. Traditional data management systems, like data warehouses, have been used for decades to store structured data and make it available for analytics. However, data warehouses aren’t set up to handle the increasing variety of data ranging from text, images, video, Internet of things (IoT), and so on, nor can they support artificial intelligence (AI) and ML algorithms that require direct access to data.
The benefits of the Data Lakehouse are:
Create value from data
Simplify your data landscape
Protect and secure your data
Scalable Analytics Platform
Machine data is frequently one of the most underutilized assets in a business, despite being one of the analytics fields with the quickest growth and being seen to be important. To achieve digital transformation, machine data must provide real-time insights and commercial value. Yet, using this data poses significant difficulties. The power of Scalable Analytics Platform in its ability to unlock data across all parts of the business. The data may come from a variety of sources, including applications, devices, networks, Internet of Things (IoT) sensors, and web traffic. With Splunk, organizations can aggregate data, ask questions, obtain answers, take action, and address business objectives. The resulting insights can help them identify security threats, optimize application performance, and understand customer behavior.
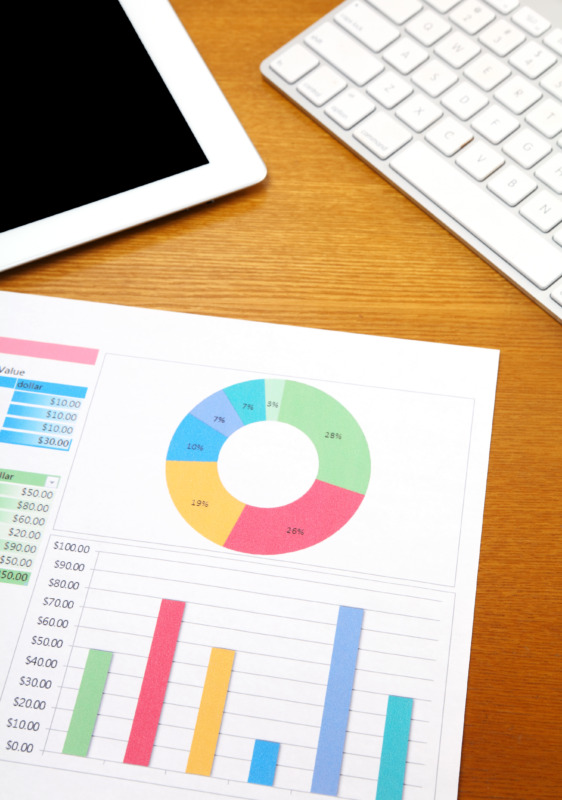
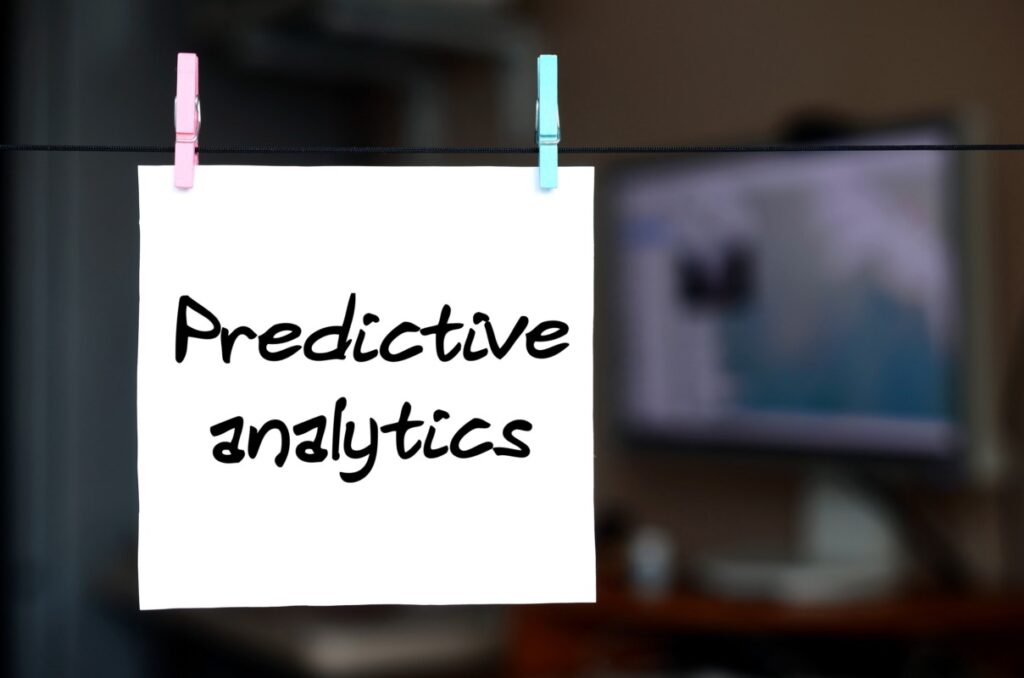
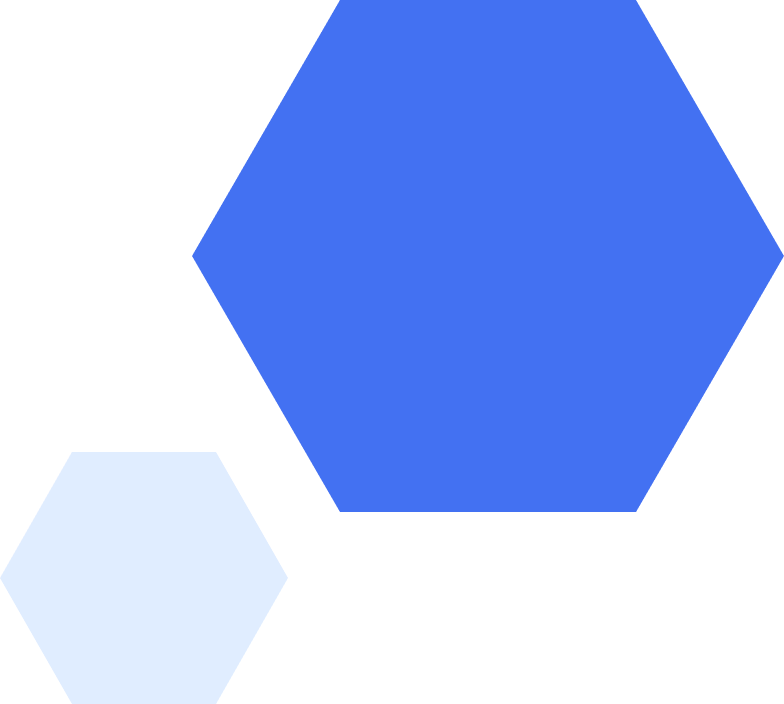
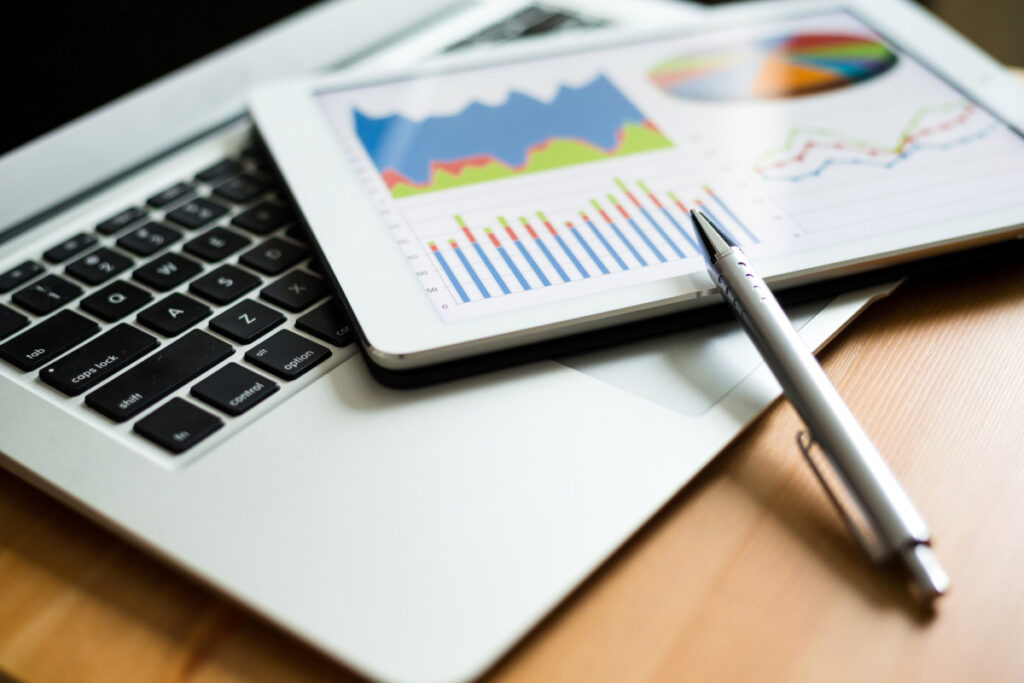
Predictive Analytic
Today’s organizations must cope with data that is being produced more quickly than ever. Industrial Internet of Things (IIoT) expansion has accelerated the number of sources, which has added to the data torrent (sensors, actuators, and other devices). The information from those sources frequently relates to the functional or operational state of connected IIoT systems or machines.
The vast amount of machine data does not always yield vast amounts of insights. One of the main reasons it is challenging to conduct studies that lead to actionable insights is the volume of current data, the speed at which new data is generated, and the variety of data created. Teams must concentrate on the when and why of unexpected, just-in-time maintenance on equipment that is going to break down and cause expensive unplanned downtime.
We can help an analytics platform that helps organizations quickly and at scale acquire visibility and insight into data to take predictive action that will benefit your business and operations.
In order to predict future events, such as the likelihood of equipment and machinery breaking down, predictive maintenance is an approach that uses data analytics and machine learning algorithms. Predictive maintenance can optimize when and how to do maintenance on IT or industrial machine assets using data, analytics, machine learning, and modeling.
The Dell Validated Design for Analytics
we can help Predictive Maintenance with combines data, statistics, machine learning (ML), and modeling to provide businesses with actionable insights about production machinery. This allows businesses to avoid making unnecessary repairs, proactively prevent potentially catastrophic machine failures, and eliminate the costs of unplanned downtime that reduce profits.
Edge-based Analytics
The ingesting, moving, processing, and storing of data incurs incremental costs that are sizeable for any project with the potential for significant return on investment. In a modern industrial facility, disk storage appliances may fill up before they could be bought and installed if data were streamed from all sources from every piece of equipment and sensor. But to what end? In the context of Industry 4.0, it is improbable that building a completely populated data lake will result in a profitable return on investment (ROI).
With this knowledge, it is possible to define data curation, which is possibly Industry 4.0’s toughest problem. How can a company sort through the sea of data presently available to find the streams of data that would aid in generating a greater ROI? The potential topics include, among others, lowering costs, lowering risks to safety, and increasing production. Nevertheless, the majority of enterprises have yet to discover an effective way to transfer data from the enormous population of industrial Internet “things” to a location where the appropriate personnel and equipment can be used to extract valuable insights.
Two more steps in the value chain need to be implemented after the problem of data curation is overcome. What techniques and technologies are necessary to help data scientists and software programmers build intelligent cyber-physical systems based on the selected data? Finally, how can the designers deploy their models and software close to the data sources that will supply inference and decision support? This reference architecture provides answers to these important problems by beginning with the presumption that data is accessible and affordable.
In a setting as complex as Industry 4.0, there are no magic solutions. Hence, there isn’t a single technology or approach that can overcome the difficulties in designing, developing, and implementing cyber-physical systems for every type of issue. The finest solutions are created with technology that can be used for several use cases and applications.
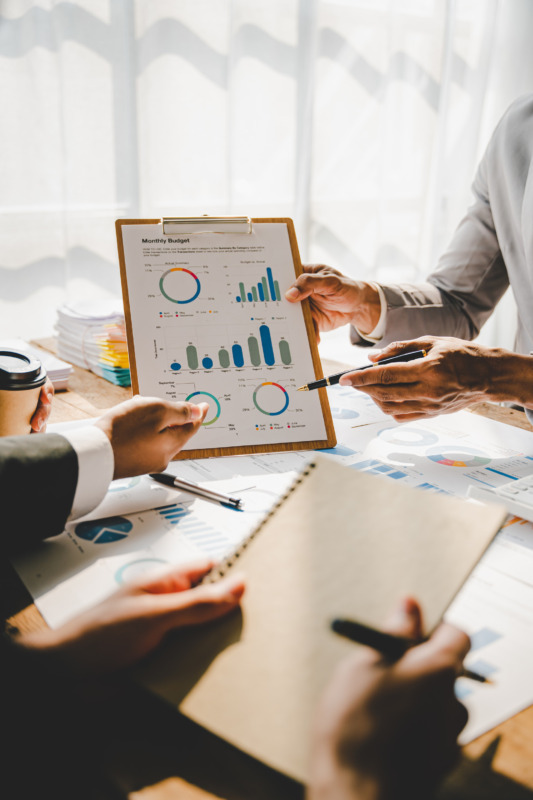
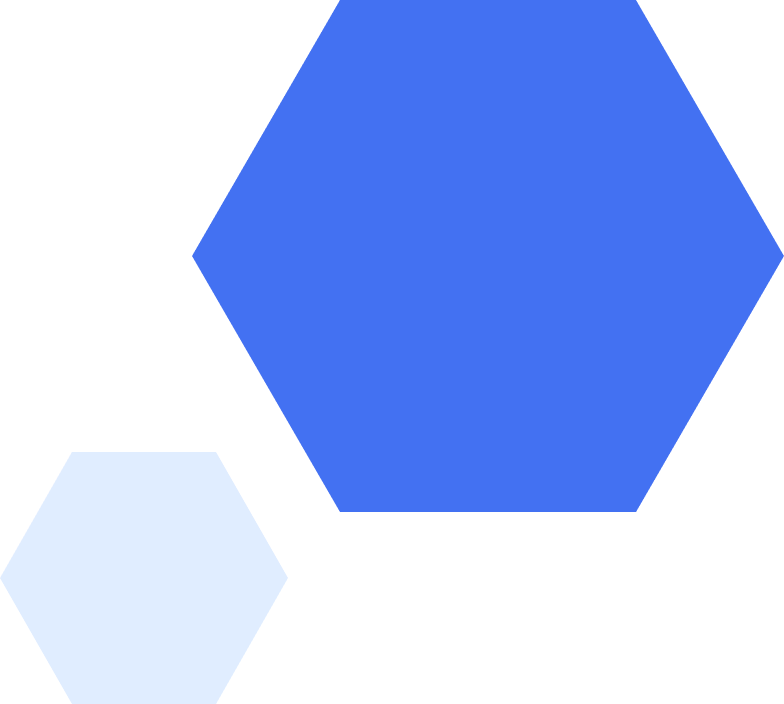
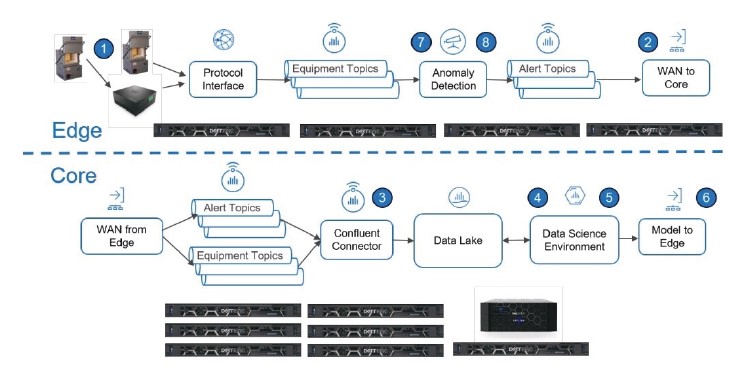
Industry 4.0 analytics methods must dynamically choose and route the necessary application data from a subset of devices. This data must then pass through processing upstream, at a core, or in the cloud. Industry 4.0 practitioners need to be able to manage data flows as well as flow data model artifacts to infrastructure that supports practical, problem-solving applications like increasing equipment reliability, controlling worker health and safety, and boosting yields.
The Dell Technologies Validated Design for Analytics
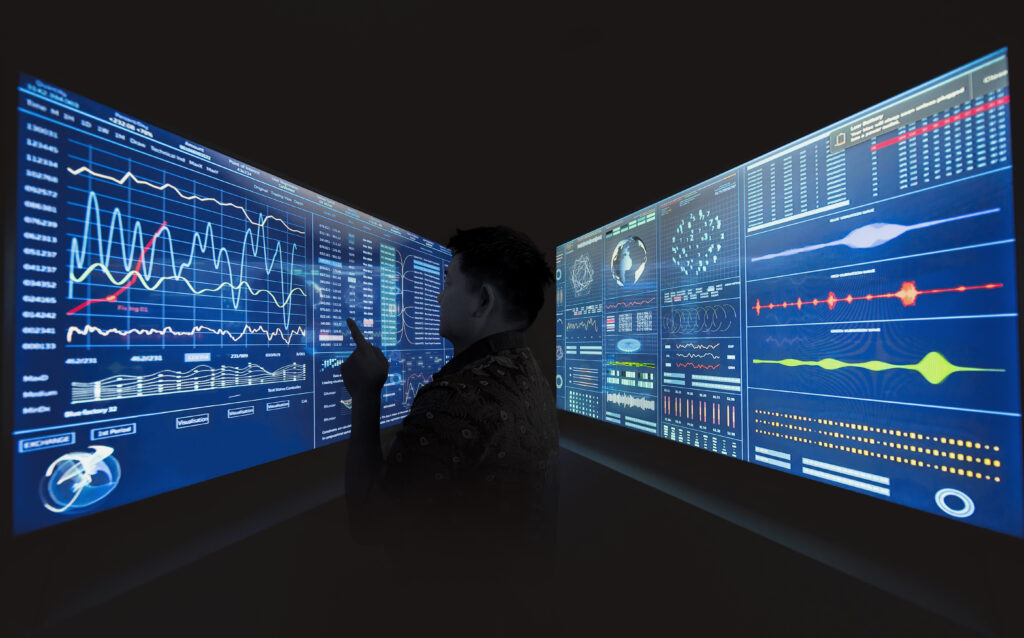
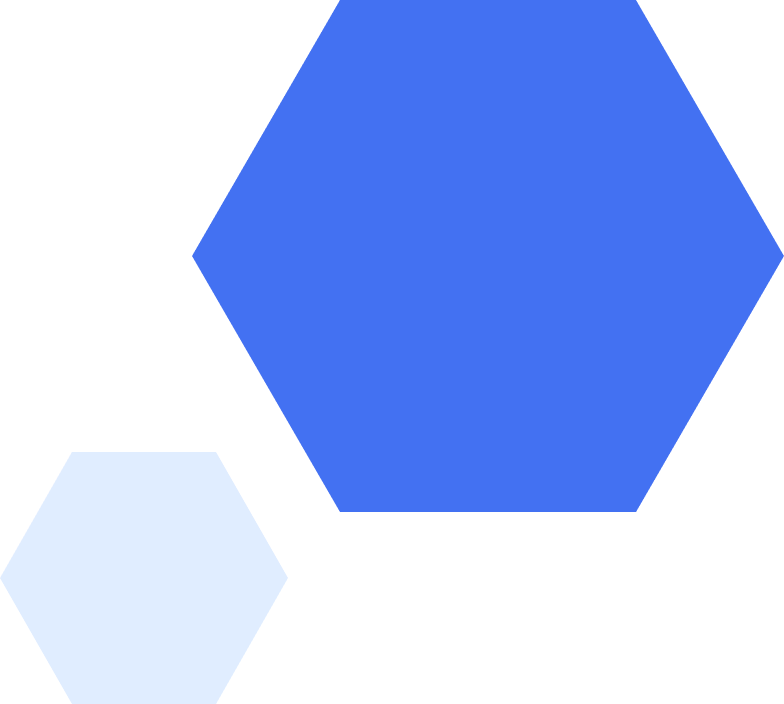
Real-Time Data Streaming
In order to foster innovation, boost agility, and assist the development of new digital products and services, businesses are updating their IT architecture. As businesses move from batch processing to real-time data and event-driven applications, these changes are also causing a fundamental change in how data supports a company’s core business.
A data streaming platform is the first step on the road to becoming a real-time enterprise. A streaming platform combines a storage layer and a processing layer with the publish-subscribe model’s strengths in data delivery. As a result, setting up data pipelines and connecting them to all of your systems is much simpler. By implementing a streaming platform, apps that require data can access it instantly without experiencing the latency of batch processing.
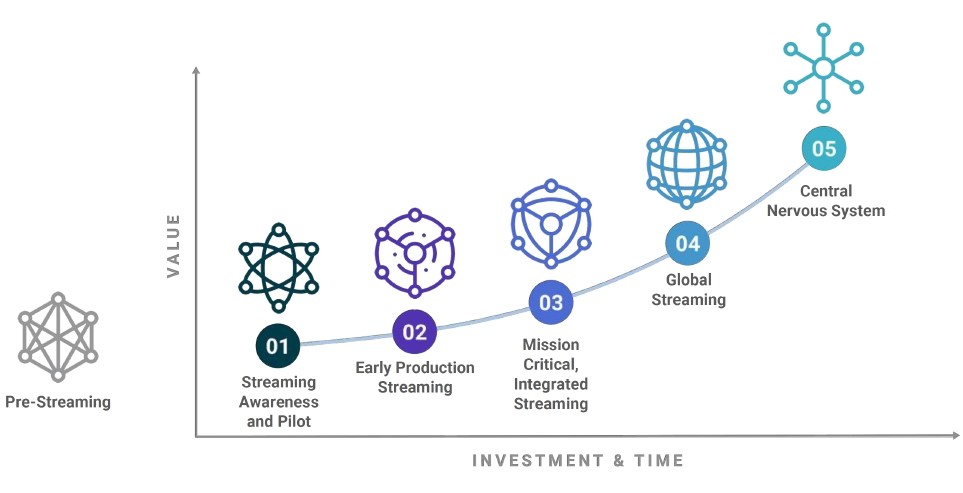
Figure 1: Streaming adoption journey illustrates the logical development of streaming data analytics adoption in the majority of client environments. The success, performance, and scalability of a streaming platform depend on selecting the right infrastructure.
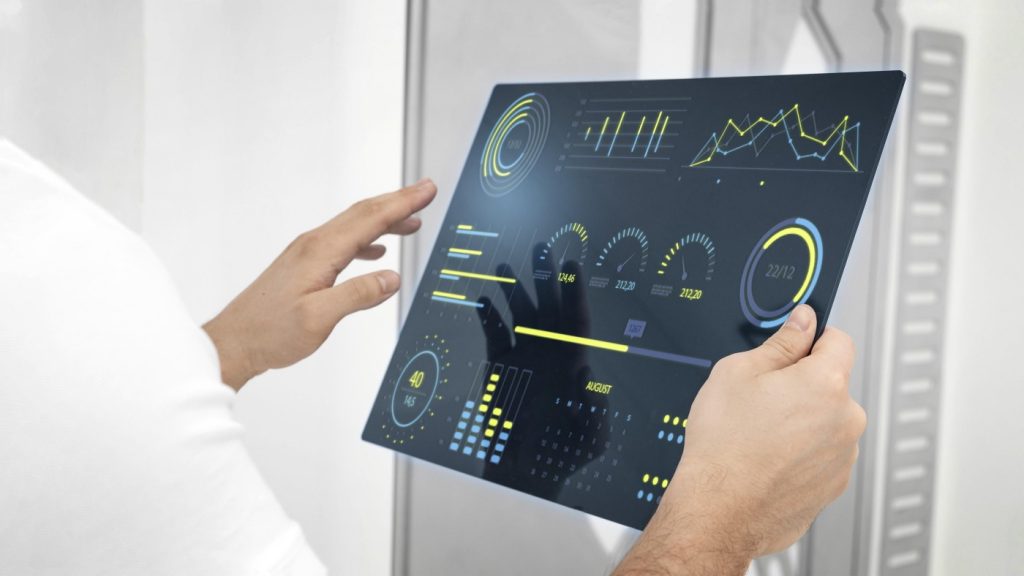
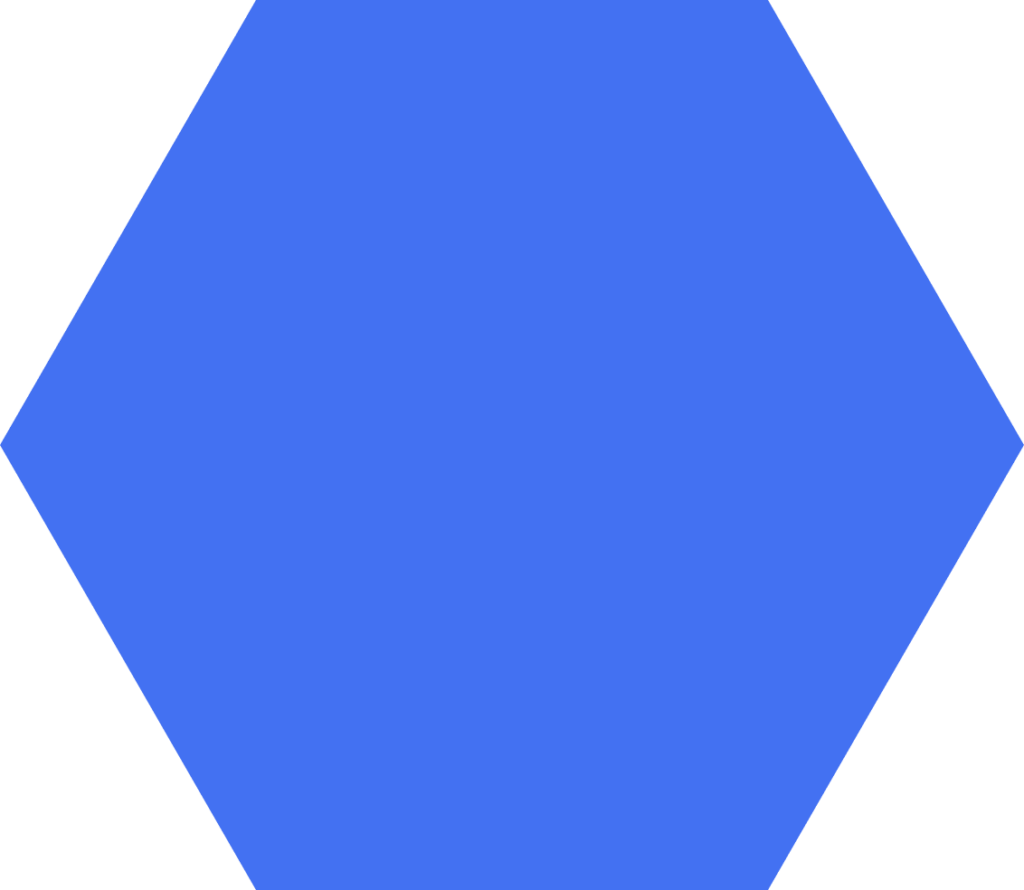
The benefits gained from using the Validated Design for Real-Time Data Streaming are:
Large-Scale Streaming Data Processing
For enterprises to use analytics to improve business decisions and power the next generation of machine learning (ML) applications, they need to be able to process vast amounts of data, whether it be batch or streaming data.
In the meantime, data science has grown beyond the capabilities of IT operations specialists, scaling vast, dispersed systems to meet the needs of data scientists working with growing data volumes offers new obstacles. Kubernetes significantly contributes to this area.
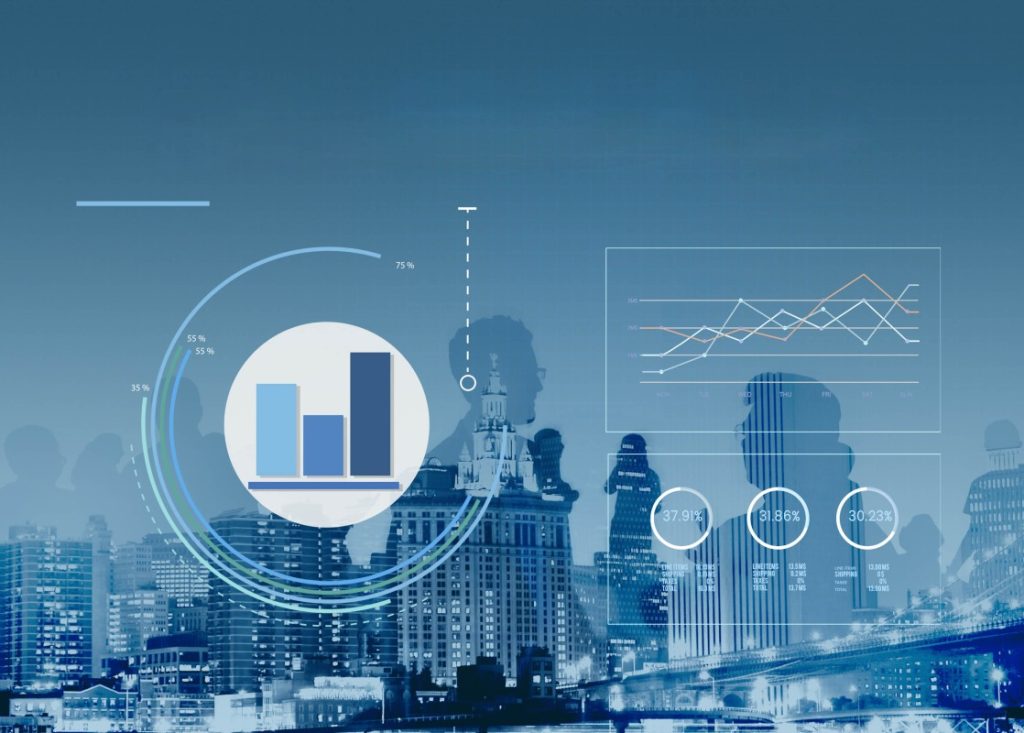
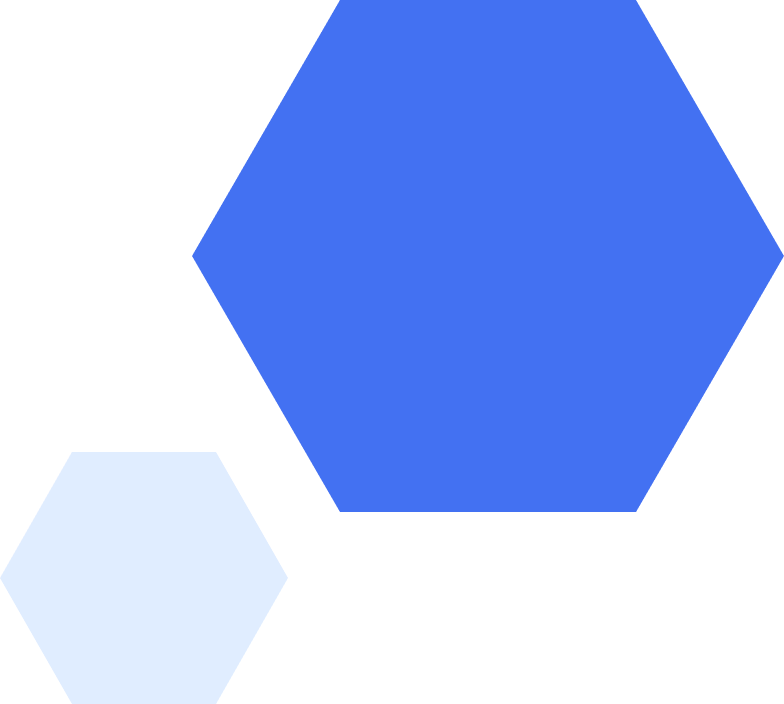